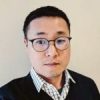
Dr.
Park, Yongjin
MSc, PhD
e-mail:
Lab Website
Academic Rank:
Assistant Professor, Dept of Pathology & Laboratory Medicine
Affiliation(s):
BC Cancer Research Centre
Location:
BC Cancer Research Centre
Short Bio
Dr. Yongjin Park is a principal investigator (Scientist) in the Department of Molecular Oncology at BC Cancer Research, part of the Provincial Health Services Authority. Dr. Park earned his Bachelors of Science in Biology and Computer science and Engineering from Seoul National University in South Korea in 2006. His Interests in biology and computer science in his undergraduate degree lead him to a Masters of Science in Computational Biology from Carnegie Mellon University in Pennsylvania graduating in 2008. From there Dr. Park ventured to Maryland where he earned his PhD in the number one ranked Biomedical Engineering program in the U.S. from Johns Hopkins University in 2014. He completed his post doctorate at Massachusetts Institute of Technology’s Computer Science and Artificial Intelligence Laboratory, and remained there until Dr. Park joined us at BC Cancer.
He is also an Assistant professor in the Department of Pathology & Laboratory Medicine and the Department of Statistics at the University of British Columbia (UBC). He leads an interdisciplinary team of young scholars consisting of statisticians, computer scientists, and bioinformaticians. His research primarily focuses on developing scalable probabilistic inference methods to elucidate hidden causal mechanisms of human diseases, such as cancer and other common/complex disorders.
Current Openings & Opportunities
Current Projects In My Lab include
Research
Our lab in BC Cancer Research conducts causal inference research to understand the mechanisms of complex disease development and progression using machine learning and data science. We develop causal inference and machine learning methods widely applicable in single-cell genomics, multi-omics data integration, and statistical genetics research. We apply our computational methods to large regulatory genomics and single-cell transcriptomics data generated by international consortia, such as NIH ENCODE and GTEx, Human Cell Atlas, and Tabula Muris/Sapiens (Chan-Zuckerberg BioHub). We are also privileged to contribute to single-cell data analysis in collaborations locally with the experimental labs at BC Cancer Research Institute, including Dr. Samuel Aparicio, Dr. Poul Sorensen, and Dr. Ramon Klein Geltink.
Our lab is uniquely situated across multiple disciplines: single-cell genomics, statistical genetics, causal inference and machine learning. Through this vantage point, not only do we push forward the frontiers of machine learning research in biology, but our research program also seeks to identify uncharted directions in computational biology research. Our computational research involves reproducible, efficient, and widely-applicable software development. We have rich experience in scientific computing and machine learning before and after the deep learning renaissance. If needed, instead of being confined by existing tools and libraries, our computational team can initiate research from a low-level programming language